Generative AI in the Newsroom: The Future of Media and News
- Dec 31, 2023
- 13 min read
This article includes insights and learnings from an applied workshop that I led with a group of European journalists in national public radio and broadcasting on “Generative AI in the newsroom,” which took place on September 29, 2023, at the UC Berkeley Graduate School of Journalism.
As futurists like to emphasize, looking back is a fundamental exercise in identifying patterns, detecting emerging trends, and anticipating what comes ahead. In the case of GenAI (Generative Artificial Intelligence), looking back 60 years and zooming in on the last year has been a particularly exciting (and overwhelming) exercise for the futurist community.
Looking Back: The 70-year Time Window; the 10-month Time Window
After the early developments of artificial intelligence in the 1950s – mostly around game-solving at first – and the emergence of GenAI (Generative Artificial Intelligence) in the 1960s, we had to wait for significant increases in computer processing capacity to notice the scaling of GenAI. Today, AI in its many diverse forms integrates with a range of business tools to serve commercial and industrial applications, from recommendation engines and customer service chatbots, to conversations with your home assistant and even protein and molecule creation.
We saw GenAI gain in importance in 2014 with the development of GANs (Generative adversarial networks). Interestingly enough, even if Google has been a bit quiet these past pandemic years, and the GenAI popularization in 2022 has been ignited by OpenAI, GenAI has been largely enabled by Google Brain, which introduced the Transformer in 2017 and accelerated with LLMs (Large Languages Models) and BERT in 2018. The acronym GPT (Generative Pre-trained Transformer) describes a series of LLMs developed by Open AI. GPT3 was the third generation, released in 2020, and GPT4 was more recently launched in March 2023.
The most important date that triggered an acute democratization of GenAI is November 30, 2022, when OpenAI launched a user-friendly version of its LLM, namely ChatGPT. Since then, the world has been through an acceleration of events. I captured those consequent signals of change in a timeline, which I invite you to consult here.
The succession of events over the past 10-11 months clearly points out that we are at an inflection point in history, as the future force emerges, with GenAI impacting our environment on all STEEPLE dimensions (social, technological, economic, environmental, political, legal, and ethical).
The 10-month Gen AI Revolution and the Rise of the Identity Economy
Synthesizing those hundred signals into an extract of ten main trends helps to alleviate the profusion of changes.
We notice that transformation is happening at a plurality of levels – product innovation, human behaviors, business-use cases, workplace adaptation, creative endeavors, impact on society, and regulatory considerations. On the cultural and semantic level, we witness the emergence of new defining concepts such as hallucinations, prompting, prompt engineering, prompt hacking, unlearning, uncropping, inpainting, outpainting, and probably a few others that will be created between today, as I write this article, and the day of publication. All industries, all job functions, all activities of human life are more or less impacted, and it seems like none will be spared.
Some functions and industries such as Marketing and Technology are leading the way, while others are lagging behind or starting to experiment with more general use cases far from the core business. The Communications and Media industry is closer to average according to Accenture’s AI maturity index, but it poses specific challenges, being an industry strongly relying on content generation -- on writing, composing, acting, and creating as tools for the core business. The news industry subsegment faces the additional challenges of “truth management” and trust building with its audience.
INSIGHTS FROM THE 10-MONTH GEN AI REVOLUTION
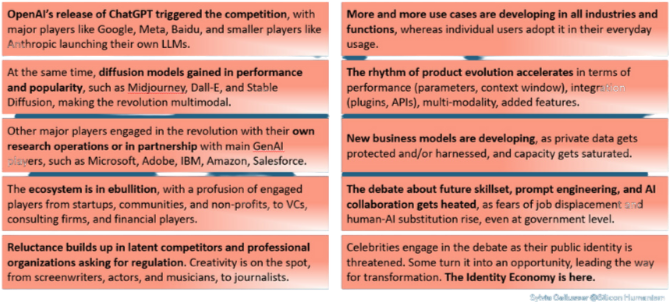
Beyond the hype, what is at stake for journalists?
Within the news industry and the journalistic profession, the debate is intense and passionate as it relates to core values of democracy, with an implicit call-to-action to continue to protect the freedom of the press. We propose to disentangle this debate into eight sub-topics.
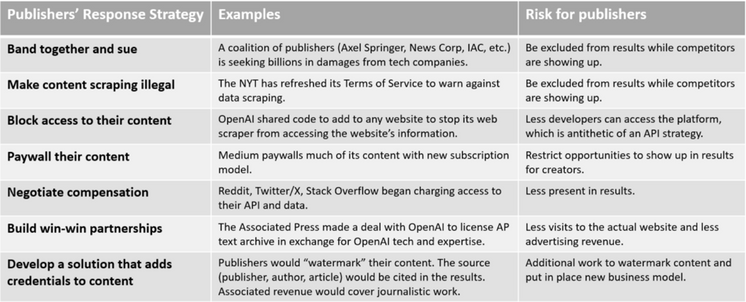
Debate #1: Scraping Journalists’ Work
This past summer has been tense for the news industry, in reaction to the GenAI revolution and to LLMs’ current success largely relying on the quality of their training data set, which includes content produced by journalists or social media content. Publishers have been through a range of reactions, illustrating the difficulty for media institutions to find the appropriate response strategy to GenAI tools scraping the work produced by journalists.
We now notice a convergence towards a few dominant positions – opposition (such as Axel Springer, News Corp, or the New York Times suing, blocking access to their content, and refreshing their Terms of Service to warn against data scraping), synergies and partnerships (such as the Associated Press with OpenAI), or transaction (such as Reddit and Twitter/X charging for access to their API and data).
The need for watermarking and credentialization is becoming pressing and being explored at the government level in the U.S., although no technical solution seems to be satisfying and robust enough at this stage.
Debate #2: Authorship and Copyright
Digital creator Stephen Thaler sued the U.S. Copyright Office after it rejected his application to copyright an image created by his Creativity Machine algorithm. The U.S. District Court Judge ruled that AI-generated artwork cannot be copyrighted, stating that human authorship is a prerequisite for copyright protection. In reaction, Thaler argued the image should be copyrighted with him as owner and the Creativity Machine as author. The judge maintained that non-human creators such as algorithms cannot hold a copyright, although she acknowledged approaching frontiers where artists use AI tools to create. This raises questions about how much human input is needed for AI-generated art to warrant copyright.
On August 30, 2023, the U.S. Copyright Office published a Notice of Inquiry and Request for comment in connection with its ongoing
study of the copyright law and policy issues raised by AI. The Copyright Office is exploring a wide range of issues, including whether changes are required to certain fundamental copyright principles to adapt to AI.
It is an opportunity for journalists and citizens to contribute to the democratic debate.
Celebrities begin to embrace the idea of their AI doppelgangers by taking meetings with AI startups and licensing their digital likenesses. These AI-generated versions of celebrities can then be used in various ways, from appearing in games, advertising, consumer goods, or artistic productions. It could create new opportunities for consumers to interact with their favorite stars in novel ways. By establishing “the contractual language, court decisions and ethical standards” overseeing digital doubles, celebrities may teach us how to defend our stakes in the age of AI.
Debate #3: Bias in Input, Bias in Output
Baidu had to submit security assessments to the government and prove compliance with China’s GenAI guidelines before commercially launching its chatbot Ernie. Its training data had to come from sources deemed legitimate by the government. As a consequence, Ernie's responses are limited. Ernie is unable to answer questions about human rights violations in Xinjiang and Tibet, the health of Chinese leaders, or Tiananmen. Ernie reckons that Covid-19 originated among American vape users in July 2019; then the virus was spread to Wuhan via American lobsters. It draws a blank if asked about the drawbacks of socialism and deflects sensitive questions with a "Let's talk about something else."
A recent paper claimed that ChatGPT aligns more closely with Democrat/left-wing perspectives than Republican/right-wing, but new research was unable to reproduce those findings because those models are evolving and a slight difference in the way we prompt them can produce very varied and unexpected results. The thing is political bias is extremely hard to detect and eradicate. On one hand, chatbots tend to narrow the over-tone window of what is considered acceptable speech by telling users that certain topics are off limits. On the other hand, LLMs’ responses tend to reflect a certain worldview, and subtly nudge users toward that view, which can be a serious threat especially as such tools are more and more in use in education.
The concept of inbreeding pinpointed by Louis Rosenberg highlights a rising phenomenon of qualitative human-generated content being degraded over time after multiple AI-transformations. Rosenberg notes: "From an evolutionary perspective, first generation large language models (LLMs) and other gen AI systems were trained on a relatively clean 'gene pool' of human artifacts, using massive quantities of textual, visual and audio content to represent the essence of our cultural sensibilities. (...) But as the internet gets flooded with AI-generated artifacts, there is a significant risk that new AI systems will train on datasets that include large quantities of AI-created content. This content is not direct human culture, but emulated human culture with varying levels of distortion, thereby corrupting the ‘gene pool’ through inbreeding."
Debate #4: Fake News
The Guardian newspaper was asked to identify an article that had supposedly been written by one of its reporters, but it was nowhere to be found in its records. The reporter didn’t remember writing the article because it had never been written. ChatGPT had hallucinated and created a false reference. The Guardian decided to respond by creating a working group and small engineering team focused on learning about the technology, considering the public policy and IP issues, listening to academics and practitioners, consulting and training their staff, exploring safely and responsibly how the technology performs when applied to journalistic use, and reflecting on what journalism is for, and what makes it valuable.
Misinformation experts say we are entering a new age where distinguishing what is real from what isn’t, will become increasingly difficult. OpenAI, Google, Microsoft, Amazon promised the U.S. government they would try to mitigate the harms that could be caused by their tech. There is no standard for watermarking: visible watermarks (easy to remove) like Dall-E and Firefly, metadata, pixel-level watermarks invisible to users, etc. These techniques need to be interpreted by a machine, then flagged to a user. It’s even more complex with mixed media content, such as a TikTok video aggregating audio, image, video, and text. Manipulated media is not fundamentally bad if you're making content meant to be fun and entertaining. The existence of synthetic content sows doubt about the authenticity of any content, allowing bad actors to claim that even genuine content is fake.
The symmetrical aspect of people believing in deep fake content to be real, is people not believing in facts even when content is verified. And this can be as harmful. Elon Musk's lawyers recently tried to argue that comments he made at a conference in 2016 could have been altered by AI, but the judge did not accept this argument. Experts are worried that as the technology becomes more prevalent, people may become more skeptical of real evidence. The "liar's dividend" coined by law professors Bobby Chesney and Danielle Citron in 2018 describes the idea that as people become more aware of how easy it is to fake audio and video, bad actors can weaponize that skepticism.
An AI detector developed by the University of Kansas can detect AI-generated content in academic papers with a 99% accuracy rate. OpenAI's Classifier has a success rate of 26%, while Winston AI, Copyleaks, and TurnitIn have accuracy rates in the 98%-99% range. A UC Davis student alleges she was falsely accused by her university of cheating with AI. A new paper from eight academic authors who empirically tested the performance of fourteen AI text detection systems, found the tools were neither accurate nor reliable, with most scoring below 80% accuracy. The tools were biased towards classifying text as human-written rather than detecting AI content, with 20% of AI text misclassified as human.
Alternate solutions we need to advance to mitigate the risks of AI-generated content include watermarking, education, and regulation.
Debate #5: The End of Writing
News organizations have taken different positions in regard to using AI for writing content. The AP has published AI-written financial stories since 2016 with a goal of giving reporters time to focus on in-depth reporting. The BBC has experimented with AI for local election updates. Yle (Finnish Public Service Media Company) briefly used an AI to write sports stories. CNET announced it was pausing its program to write stories using AI after resulting articles were not only riddled with errors, but rife with plagiarism. The CEO of Axel Springer SE, a German media company, wrote in a company email: “AI has the potential to make independent journalism better than it ever was — or simply replace it.”
On the side of tech companies, Google is testing an AI tool called "Genesis" that can write news stories and has pitched it to several news organizations such as The New York Times, The Washington Post and The Wall Street Journal’s owner, News Corp. Google believes the tool can serve as a personal assistant for journalists by automating some tasks (drafting headlines, writing styles, etc.) in order to free up time for others. Google considers the tool as a form of “responsible technology.” Some executives find it unsettling, concerned that AI-generated stories without fact-checking or editing have the potential to spread misinformation.
As highlighted by American journalist Jeff Jarvis, literacy is still an issue in education, and GenAI could be an enabler for democratization. He writes: "How many people today say they are intimidated setting fingers to keys for any written form because they claim not to be writers, while all the internet asks them to be is a speaker? What voices were left out of the conversation because they did not believe they were qualified to write? If the machine takes over the most drudgerous forms of writing, we humans would be left with brainpower to write more creative, thoughtful, interesting work. Maybe the machine could help improve writing overall. (...) What 'content creators' must come to realize is that value will reside not only in creation but also in conversation, in the experiences people bring and the conversations they join."
Debate #6: The Case for Translation
Last year, French daily newspaper Le Monde launched an English-language edition translated by AI. The project began when a reporter proposed writing a series of articles on the 2022 French elections in English. Given the capacity AI provided, the paper quickly shifted from a newsletter of 10-15 articles to launching an entire English-language edition covering more than 40 daily articles. Le Monde’s English posts are now written and edited in the outlet’s native language, then translated to English with AI, and reviewed by an editor before publishing. The process is called machine translation post-editing (MTPE) and it relies on a human-machine collaboration with a reduced number of collaborators.
More recently, G/O Media recently fired the staff of Gizmodo en Español and replaced their work with AI translations of English-language articles. Readers have noticed quality degradation, some translated articles switching languages midway through due to glitches in the AI translation system. The GMG Union (part of Writers Guild of America) expressed disappointment over the decision to replace the Gizmodo en Espanol team and the lack of adequate severance for the four employees who held yearly contracts with the company. The union criticized the practice of using AI tools for content production as unethical, citing factual inaccuracies in AI-generated articles. But as tools are getting more accurate, we might see more and more usage of AI in news content translation.
Debate #7: Human-AI Collaboration vs. Substitution
In other news, IBM is partnering with the Masters, Wimbledon, and US Open to provide AI-generated commentary and text captions on video highlight reels of every men’s and women’s singles match. IBM Match Insights with Watson are AI-powered fact sheets that use sophisticated data analytics and NLP to distill millions of data points into meaningful insights about each match. IBM fine-tunes its models for tennis terminology and commentary by training them on adjectives like “brilliant,” “dominant,” “impressive,” and content mined from sports blogs and articles. When using AI voices, ethical considerations are taken into account to ensure accuracy and inform listeners that what they're hearing is not a real human via disclaimers before the AI speaks. In the future, AI could enable real-time broadcast of all games and generate voices to sound like tennis stars or celebrities.
According to Canadian futurist and founder of the tech education company, WAYE, Sinead Bovell: “The most effective way to prevent cheating is to assume students are using AI and test for that knowledge in new and more challenging ways. (...) If it’s no longer sufficient for a student to write a basic paper or essay, we need to raise the bar on what humans bring to the table. (...) We did this with math. The calculator meant math got harder, so we need the same updating with AI.”
Debate #8: The Journalistic Process
As a matter of fact, publishers already use AI to craft news stories dominated by facts and figures (financial news, sports) and detect trends that could signal interesting stories. During the 2016 election, The Washington Post used its AI tool, Heliograf, to alert the newsroom when election results started trending in unexpected directions. Bloomberg’s AI tool, Cyborg, can analyze a financial report as soon as it is released, and immediately produce a news story. Thanks to automation, AP scaled up earnings reports from 300 to 3,700 articles per quarter and freed up to 20% of its reporters’ time spent covering corporate earnings. Forbes’ AI tool, Bertie, provides reporters with rough drafts and story templates.
The Radio Television Digital News Association (RTDNA) – the world's largest professional organization devoted exclusively to broadcast and digital journalism – expressed guidelines on the use of AI in journalism, highlighting questions that should guide journalists in their decision-making. It highlights the importance to be aware of the policies, guidelines, and code of ethics of the news organization and of the Generate AI tools used as they may vary.
More generally, guidelines suggest digging into archives and leveraging GenAI as a new way to interact with information and data that are built in the article or the report. Journalists are invited to promote responsible GenAI and focus on automation tasks rather than creative, and to retain robust oversight and input across all of the processes, no matter how much (or how little) GenAI tools are involved in the process. One of the most important aspects is to favor transparency by disclaiming and letting the audience know when and where the news provider is using AI, with the best interest of the audience at heart at all times. Finally, as journalists get comfortable using AI, they are encouraged to provide feedback to the news organization and to push forward best practices for the organization and other individual contributors.
Three scenarios: Frugal AI, The Tech-supercharged World, The Reality Continuum
The revolution at play in journalism and media is a fascinating lens to investigate the impact of GenAI on society at a larger scale. On the basis of this signal scanning, trend analysis, and debate investigations we started building three scenarios – Frugal AI (a constraint “for good” scenario), the Tech-supercharged world (on the brink of collapsing), and The Reality Continuum (a growth-transformation scenario) – that can serve as useful tools in ulterior foresight work. I introduce these scenarios in a series of upcoming podcast episodes by Informing Choices: “The Future of Media and News – The Role of Generative AI”. We invite you to join us in envisioning them further.
About the Author
Sylvia Gallusser is a Global Futurist based in Silicon Valley. She is a Lead Business Strategist (Metaverse Continuum Business Group) with Accenture. In addition, Sylvia is the Founder & CEO of Silicon Humanism and the host of our “Ethics of Futures” think tank within the Association of Professional Futurists (APF). Sylvia conducts foresight projects on the future of health, well-aging, and social interaction, the future of work and life-long learning, as well as transformations in mobility and retail. She is involved in the future of our oceans as a mentor at SOA (Sustainable Ocean Alliance). She closely monitors the future of the mind and transhumanism, and investigates AGI (Artificial General Intelligence), Generative AI, and AI ethics. Sylvia is a published author of Speculative Fiction with Fast Future Publishing. She regularly gives keynotes and interviews as a distinguished female futurist (keynote speaker of the latest Microsoft Elevating You conference), and teaches in MBAs, Master in Entrepreneurship (HEC Paris), and Executive programs (UC Berkeley Graduate School of Journalism).
Comments